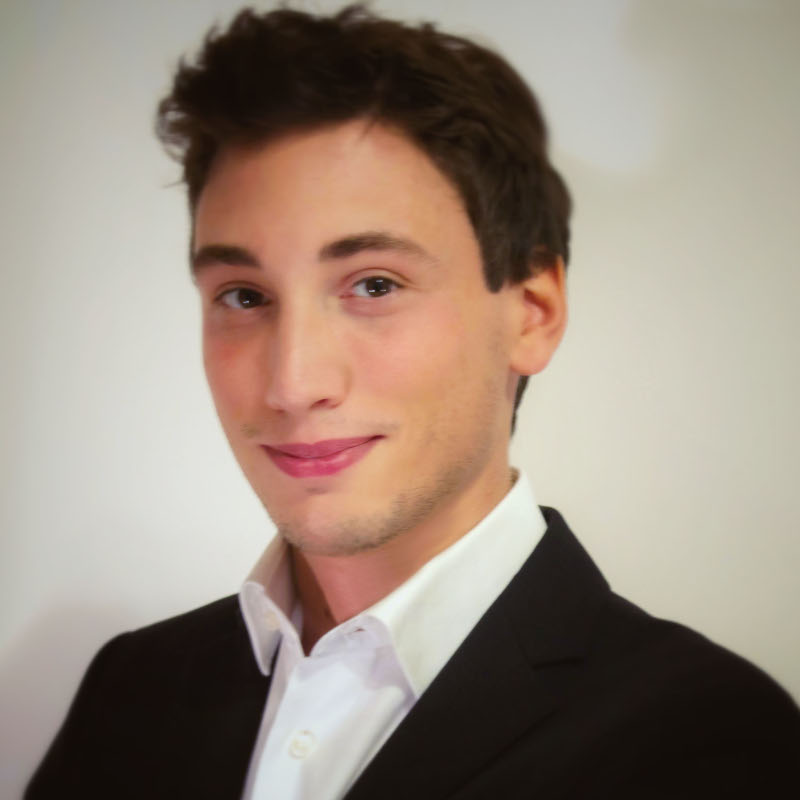
Nicola Piovesan is a Senior Researcher at Huawei Technologies, in Paris, France. His research activity focuses on large-scale network modeling, data-driven network optimization, green networking, and the integration of artificial intelligence in the telecom domain.
He earned his PhD degree in Network Engineering at the Universitat Politècnica de Catalunya (UPC), Barcelona, Spain, in 2020, and he received the BSc degree in Information Engineering and the MSc in Telecommunication Engineering from the University of Padova, Italy, in 2013 and 2016, respectively.
From 2016 to 2019, he was an Assistant Researcher at the Mobile Networks Department of the Centre Tecnològic de Telecomunicacions de Catalunya (CTTC). In 2016, he has been awarded with a European Commission’s Marie Skłodowska-Curie fellowship to work as early-stage researcher in the EU H2020 MSCA SCAVENGE (Sustainable Cellular Networks Harvesting Ambient Energy) project.
In 2019, he was at Nokia Bell Labs as a visiting researcher in the Small Cells Research Department.
Dr. Piovesan has contributed to multiple industrial and academic projects, published over 40 research articles, and is co-inventor of more than 10 patents. His work has been recognized with the Huawei GTS President Award (2021) and the Huawei Quality Star Award (2024), acknowledging his successful translation of research into real-world product development. He is actively involved in collaborative initiatives on AI and energy efficiency in next-generation networks.
Recent Publications
See allTeleMath: A Benchmark for Large Language Models in Telecom Mathematical Problem Solving
PreprintSubmitted
Authors: Vincenzo Colle, Mohamed Sana, Nicola Piovesan, Antonio De Domenico, Fadhel Ayed, Merouane Debbah
Waste Factor and Waste Figure: A Unified Theory for Modeling and Analyzing Wasted Power in Radio Access Networks for Improved Sustainability
JournalIEEE Open Journal of the Communications Society
Authors: Theodore S. Rappaport, Mingjun Ying, Nicola Piovesan, Antonio De Domenico, Dipankar Shakya
Selected Talks
See allUpcoming Talks & Events
Advancing Network Automation through Multi-Agent Large Language Models
AI for Good Summit
The Role of AI and Large Language Models in Future Wireless Networks
Cuenca Summer School
Recent Patents
See allA device and method for evaluating generative artificial intelligence models
FiledPCT/EP2025/063678 • Filed: May 19, 2025
Inventors: N. Piovesan, A. De Domenico, M. Sana, F. Ayed
Devices and methods for energy saving and energy source scheduling in cellular networks
FiledPCT/CN2025/076717 • Filed: Feb 10, 2025
Inventors: N. Piovesan, A. De Domenico, L. Madier, X. Li
Other Activities
Awards
IEEE ICC Best Paper Award
2025
Huawei Quality Star Award
2023
Huawei GTS President Award
2021